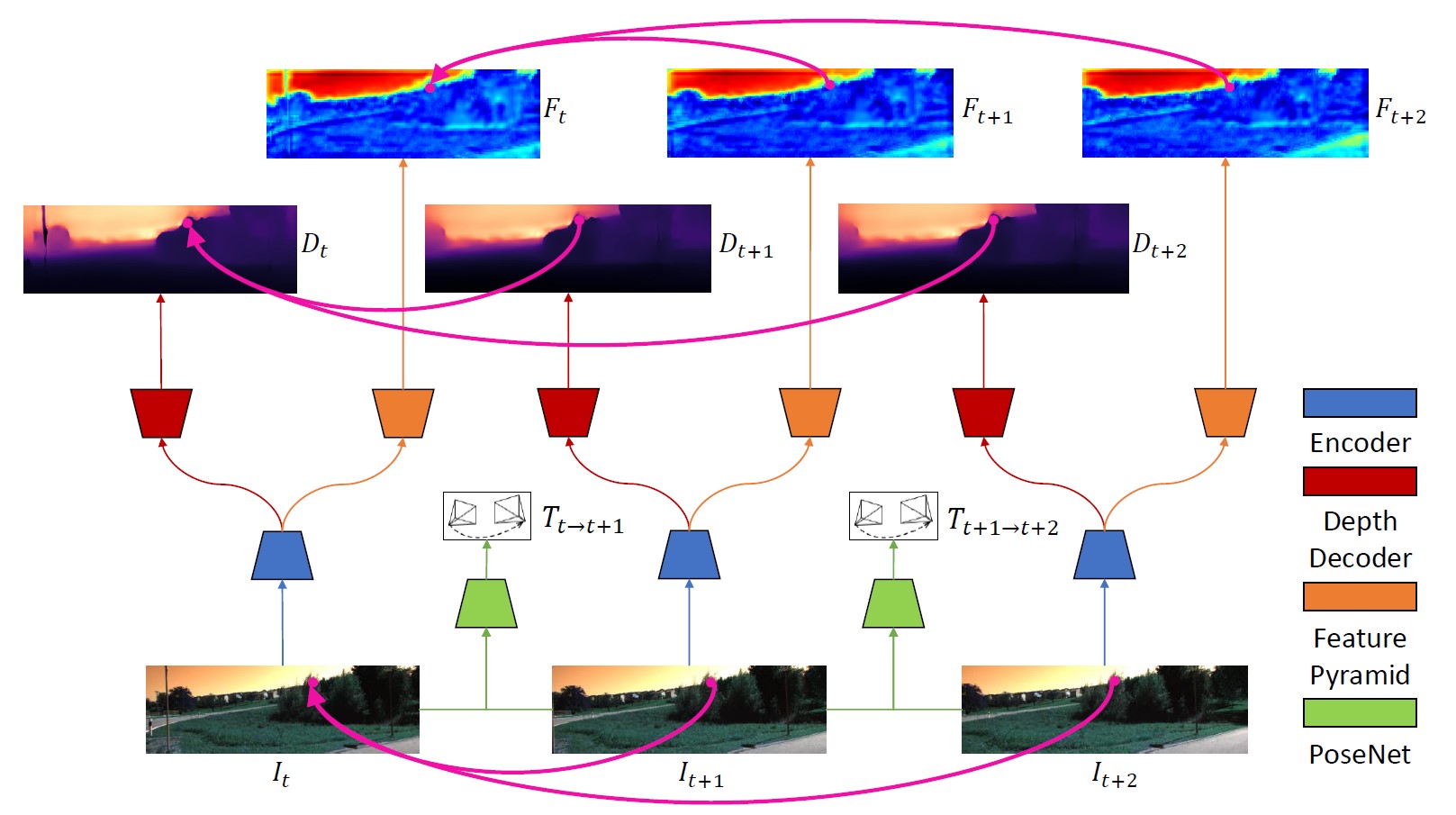
Bundle adjustment refines scene geometry and relative camera poses simultaneously via reprojection error, computed by a set of images from different viewpoints, which is the gold standard for visual odometry. However, deep learning methods have not been well exploited within this area of study. We consider that incorporating bundle adjustment into the training stage of deep learning can improve the accuracy of trained neural networks while maintaining the efficiency.
This research introduces a self-supervised learning framework for monocular visual odometry, inside which depth maps, relative camera poses, and dense feature maps (with the same resolution as images) are estimated and used for photometric, geometric, and feature-metric losses in bundle adjustment. In this manner, we consider that the learning of neural networks can be geometrically constrained by multi-view geometry. Furthermore, bundle adjustment is only required during the training time, allowing the networks to benefit from bundle adjustment without any additional computation burden during the inference time. It achieves state-of-the-art performance on visual odometry estimation at 894 FPS on RTX 3090 GPU.
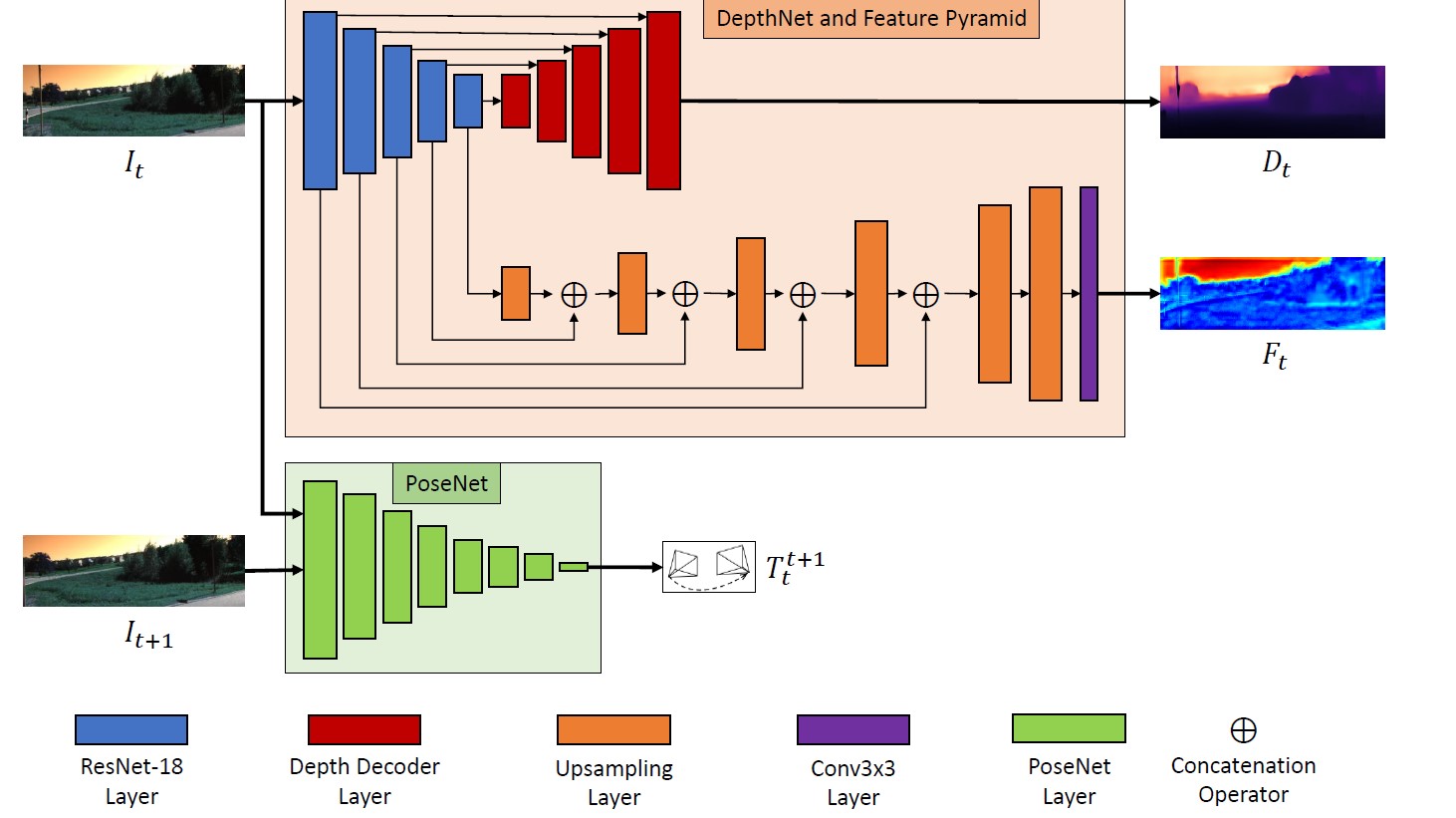
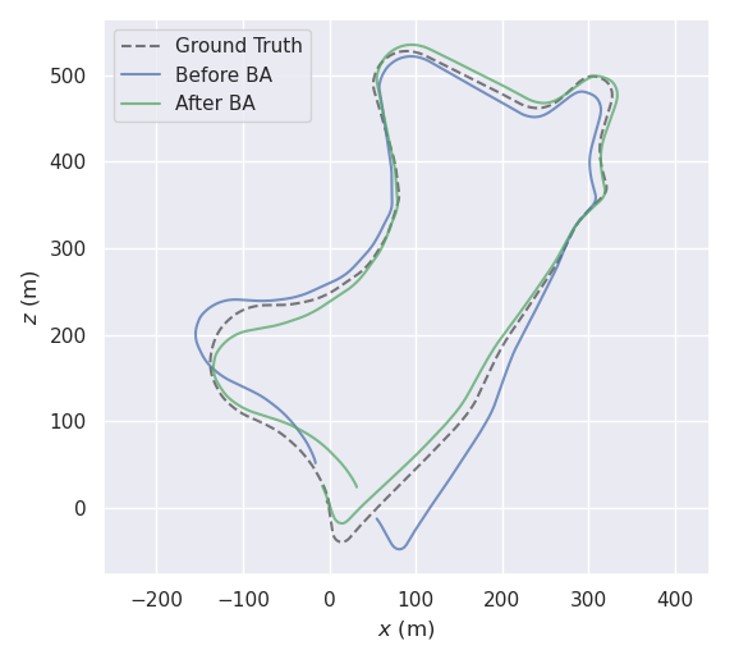
References
- Weijun Mai and Yoshihiro Watanabe: Feature-Aided Bundle Adjustment Learning Framework for Self-Supervised Monocular Visual Odometry, 2021 IEEE/RSJ International Conference on Intelligent Robots and Systems (IROS) September 27 - October 1, 2021. Prague, Czech Republic